Using Eye Tracking to Measure Implicit Learning in Students with LD, ADHD, and ASD (2017)
Description
Many students with LD, ADHD, and ASD tend to struggle in STEM classrooms. Often their struggles are rooted in Executive Function (EF) and language processing difficulties. Advances in data mining techniques that allow for nonintrusive measures of implicit learning are being explored by this project to develop a prototype adaptive version of a science learning game that can help diverse learners improve their understanding of core science concepts.
Our collaborative research team including researchers at TERC and MIT is collecting click stream and eye movement data for students playing an existing particle simulator game that has been shown to be predictive of learning outcomes. Previous work done by the EdGE team at TERC shows that students who show sound particle differentiation in their gameplay patterns tend to perform better on standard Newtonian physics items. Students who do not exhibit this is implicit knowledge in their gameplay pattern correspondingly don’t exhibit similar performance gains. By analyzing the game log and the eye movement patterns synchronized down to the millisecond level we are able to look for differences in attention allocation that are indicative of different student learning trajectories. This in turn will be used to create an adaptive prototype of the game able to strategically guide student attention in order to maximize learning.
NSF Award: 1417456
Discussion
This discussion took place during the TERC Video Showcase Event Nov. 14-21, 2023. Discussion is now closed.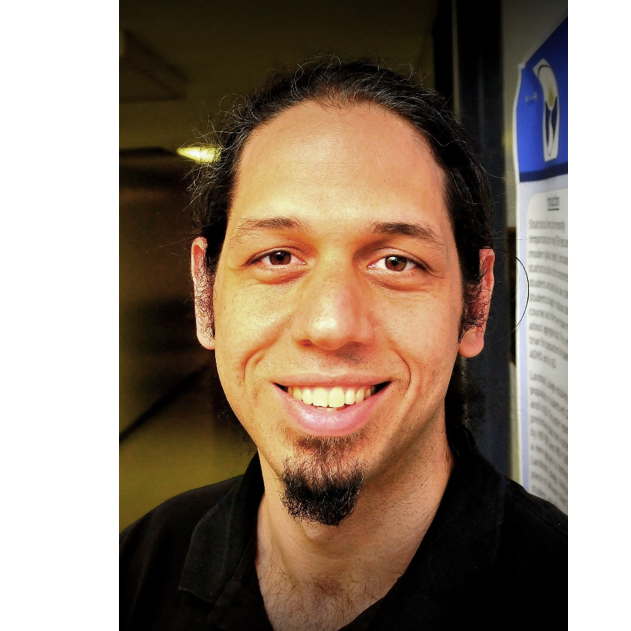
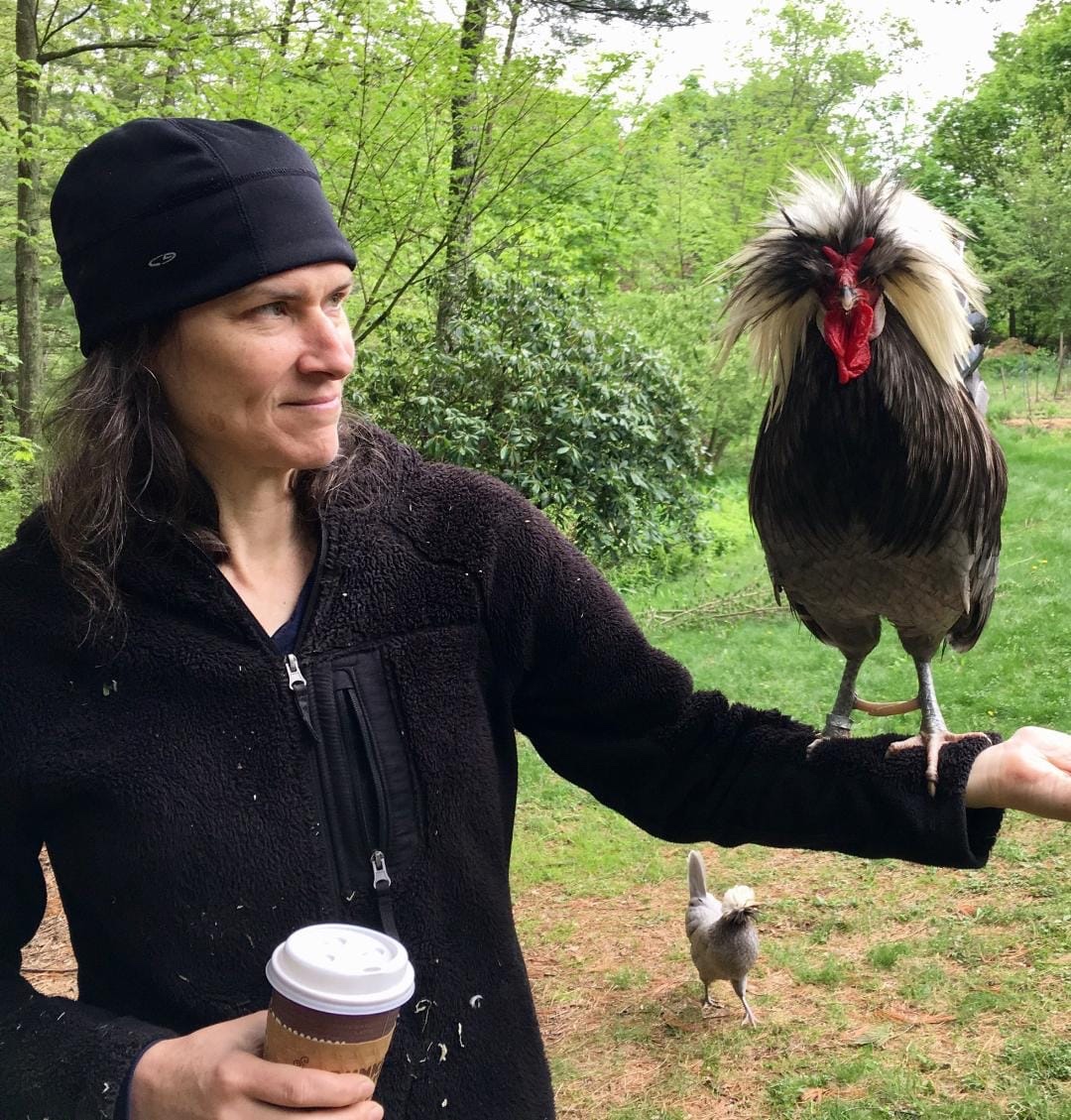
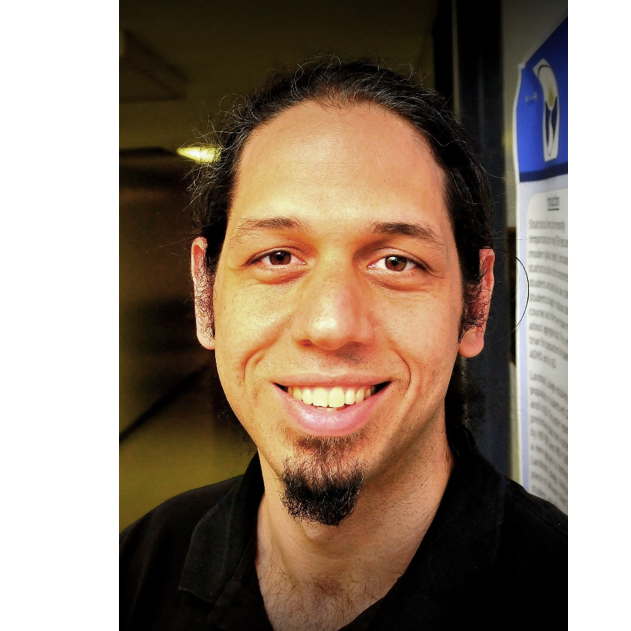