The Civic Data Project will share research as it is published about the activities in this project. Below we provide related research published by members of our team and Advisory Board.
Project Spotlight: Exploring Data Science Through the Lens of Civics Education
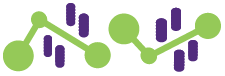
Shreiner, T. (2024) Teaching Data Literacy in Social Studies: Principles and Practices to Support Historical Thinking and Civic Engagement. Teachers College Press.
Description: We are surrounded by data and data visualizations in our everyday lives. To help ensure that students can critically evaluate data—and use it to promote social justice—this book outlines principles and practices for teaching data literacy as part of social studies education. The author shows how social studies content and skills can enhance both data literacy and its importance in supporting students’ historical thinking and civic engagement. Shreiner also provides a rationale for including data literacy in the social studies curriculum and highlights the special knowledge and skills social studies teachers offer in promoting a critical, humanistic form of data literacy. Recognizing that many social studies teachers feel poorly equipped to teach data literacy, this book offers practical advice, summaries of the benefits and challenges to students, guidance for incorporating data literacy across elementary and secondary grades, and strategies to help students analyze, use, and create data visualizations.
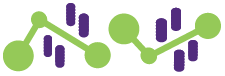
Miller, Katherine M., et. al. (2024). Data and Social Worlds: How Data Science Education Supports Civic Participation and Social Discourse. International Society of the Learning Sciences (ISLS) Annual Meeting
Abstract: As the world becomes increasingly awash in data, the ability to engage in civic participation and social discourse is becoming more dependent on the ability to engage with large real-world data sets. Data science education, as a growing focus across disciplines and age groups, strives to prepare learners to be active citizens by supporting them in engaging in inquiry with data that intersects with social and civic phenomena. The eleven projects represented in this symposium explore how learners across age groups and geographic locations are supported for civic engagement and the examination of social worlds through data-based inquiry. The projects take place in an eclectic and diverse range of settings and disciplines, tied together through the convergence of data science education and civic and social engagement. Two projects from this symposium:
Exploring data through the lens of the United Nations Sustainable Development goals: What actions can youth take? Andee Rubin & Traci Higgins
The Data Explorers program, funded by NetApp as part of its Corporate Social Responsibility program, engages middle school-aged youth around the world in exploring data related to the United Nations Sustainable Development Goals. The program has an explicit goal of inspiring participants to connect data to action. Several design decisions were adopted to support students in making this connection. The poster describes several key design decisions and how those decisions helped us create authentic opportunities for youth to connect data to action.
Investigating large, authentic, complex datasets at DataFest: How the goal of communicating actionable insights shapes the process. Traci Higgins, Jessica M. Karch, & James K.L. Hammerman
This research explores how teams of undergraduate students leverage multidisciplinary formal and informal knowledge, skills, and perspectives to navigate being awash in large, authentic, complex (LAC) data at DataFest (DF), a co-curricular, hackathon-style competition. An emerging finding from our mixed methods research is that teams draw on a broad range of knowledge sources beyond the domains of statistics, the computational sciences, and the discipline from which the data came from to gain traction in framing their investigation, identifying actionable insights, and communicating findings. Although we often think of analysis as central to teaching data science, it is the framing, search for actionable insights, and communicative intent that helps students resolve feeling awash in LAC and engage productively in the investigative process.
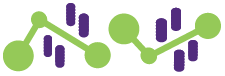
Higgins, T., Mokros, J., Rubin, A., Sagrans, J. (2023). Students’ approaches to exploring the relationship between categorical variables. Teaching Statistics, 45(S1), S52-S66.
Abstract: In the context of an afterschool program in which students explore relatively large authentic datasets, we investigated how 11- to 14-year old students worked with categorical variables. During the program, students learned to use the Common Online Data Analysis Platform (CODAP), a statistical analysis platform specifically designed for middle and high school students, to create and interpret graphs. Following the program, we conducted individual clinical interviews, during which students used CODAP to answer questions about relationships between variables. Here, we describe how students engaged in exploratory data analysis that involved looking at relationships between two categorical variables. Students worked from data in table form and created “contingency graphs,” a variant of contingency tables, which they used to analyze and draw insights from the data. Our research identified four strategies that students used to examine the data in order to explore patterns, make comparisons, and answer questions with the data.
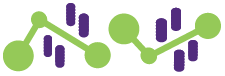
Martell, C. C., & Stevens, K. M. (2020). Teaching History for Justice: Centering Activism in Students’ Study of the Past. Teachers College Press.
Description: Learn how to enact justice-oriented pedagogy and foster students’ critical engagement in today’s history classroom. Over the past 2 decades, various scholars have rightfully argued that we need to teach students to “think like a historian” or “think like a democratic citizen.” In this book, the authors advocate for cultivating activist thinking in the history classroom. Teachers can use Teaching History for Justice to show students how activism was used in the past to seek justice, how past social movements connect to the present, and how democratic tools can be used to change society. The first section examines the theoretical and research foundation for “thinking like an activist” and outlines three related pedagogical concepts: social inquiry, critical multiculturalism, and transformative democratic citizenship. The second section presents vignettes based on the authors’ studies of elementary, middle, and high school history teachers who engage in justice-oriented teaching practices.
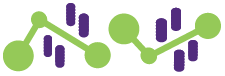
Rubin, A., Higgins, T., Mokros, J., & Sagrans, J. (2020). Data Clubs: Introducing Middle School Youth to Data Science. 2020 STEM for All Video Showcase.
Video: Data are the currency of the current era, yet most youth have not had the opportunity to develop the skills that allow them to deal productively and powerfully with data. Being “data fluent” is critical for both work and life, but data science is largely not taught at school, so informal settings are essential for filling this gap. Our project explores how we can make data science accessible to middle school youth through developing data clubs modules that are delivered in after-school programs and summer camps. Through choosing topics of interest to youth and using a highly accessible and free data analysis program, we have been able to successfully involve participants in meaty data analysis practices.
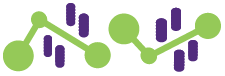
Rubin, A. (2020). Learning to reason with data: How did we get here and what do we know? Journal of the Learning Sciences, 29, 154-164.
Abstract: In “50 Years of Data Science,” Donoho claims that most of the core activities of data science are what statisticians have been doing routinely for their entire careers. Traditional statistics education, however, has primarily focused on decontextualized problems and mathematical proofs, rather than actually reasoning with data the way statisticians do. In spite of the recent uproar about how the current crop of students is totally unprepared to deal with the data deluge of the present and future, teaching students to reason with data is not a totally new enterprise, and a small but insistent statistics education community has been studying the process for decades. In this commentary, I emphasize five critical aspects of working with data that have emerged from this body of work: context, variability, aggregate, visualization, and inference. I believe these will remain relevant in spite of the addition of new techniques to our arsenal of methods for making meaning using data and can form the basis for ongoing collaborations among learning scientists, statistics educators and data science educators.
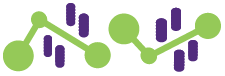
Rubin, A. & Mokros, J. (2018). Data clubs for middle school youth: Engaging young people in data science. Proceedings of the 10th International Conference on Teaching Statistics, Kyoto, Japan.
Abstract: We describe a project to engage middle school students in out-of-school Data Clubs whose goal is to give them a “taste” of data science. We identify four criteria for materials we are developing, related to topics, datasets, tools, and activities. We discuss our justification for each, provide examples of how they might be realized, and consider the complexity of satisfying all four criteria simultaneously.
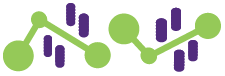
Konold, C., Higgins, T., Russell, S.J., & Khalil, K. (2015). Data Seen Through Different Lenses. Educational Studies in Mathematics, 88(3): 305-325.
Abstract: Statistical reasoning focuses on properties that belong not to individual data values but to the entire aggregate. We analyze students’ statements from three different sources to explore possible building blocks of the idea of data as aggregate and speculate on how young students go about putting these ideas together. We identify four general perspectives that students use in working with data, which in addition to an aggregate perspective include regarding data as pointers, as case values, and as classifiers. Some students seem inclined to view data from only one of these three alternative perspectives, which then influences the types of questions they ask, the data representations they generate or prefer, the interpretations they give to notions such as the average, and the conclusions they draw from the data.